The Role of Medical Datasets for Machine Learning: Transforming Healthcare
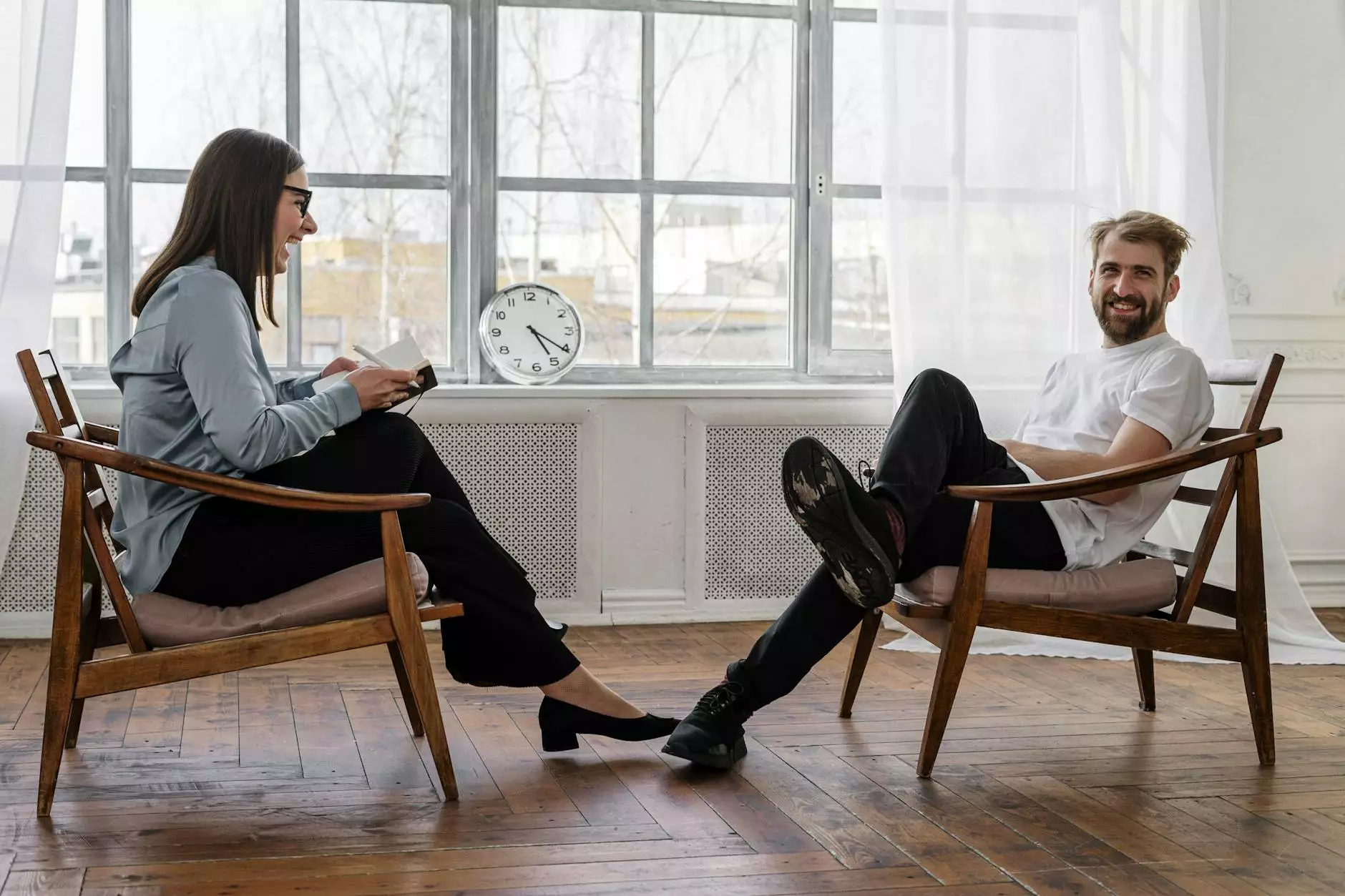
Understanding Medical Datasets in the Realm of Machine Learning
In recent years, the fields of medicine and technology have become increasingly intertwined, leading to groundbreaking advancements. One of the most significant contributions to this synergy is the emergence of medical datasets for machine learning. These datasets are foundational to developing algorithms that improve diagnosis, treatment, patient care, and outcomes.
What Are Medical Datasets?
Medical datasets comprise collections of data relevant to healthcare, which can include:
- Patient Records: Information about individual patients, including demographics, medical history, treatment plans, and outcomes.
- Clinical Trials: Data from experiments designed to evaluate the effectiveness of new treatments.
- Medical Imaging: Images such as X-rays, MRIs, and CT scans that can be analyzed by algorithms to detect anomalies.
- Genomic Data: Information related to genetic sequencing that can provide insights into disease predispositions.
The Importance of Medical Datasets for Machine Learning
Machine learning (ML) algorithms thrive on data. The rich variety and volume of medical datasets allow these algorithms to learn and make decisions. Here’s why they are crucial:
- Data-Driven Insights: Machine learning models can identify patterns and correlations in vast datasets, leading to more informed clinical decisions.
- Predictive Analytics: With sufficient data, ML can predict future health trends, patient outcomes, and potential disease outbreaks.
- Personalized Medicine: By analyzing genetic and health behavior data, machine learning can help tailor treatments to individual patients, enhancing effectiveness.
- Resource Optimization: Healthcare facilities can analyze datasets to better allocate resources, improve workflows, and reduce costs.
Challenges in Collecting Medical Datasets
Despite their value, collecting medical datasets comes with challenges:
- Data Privacy: Patient confidentiality and compliance with regulations like HIPAA make data collection and sharing complex.
- Data Quality: Inconsistent data entry practices and incomplete records can lead to unreliable datasets.
- Integration Issues: Combining data from diverse sources, such as hospitals and clinics, can be technically challenging.
How Medical Datasets Enhance Machine Learning in Healthcare
The integration of medical datasets into machine learning is transforming healthcare in multiple ways:
1. Improved Diagnostics
Machine learning algorithms can analyze medical images more accurately and quickly than human clinicians. For example, they can detect early signs of diseases like cancer through imaging data.
2. Enhanced Treatment Plans
By analyzing historical treatment data, machine learning can suggest optimal therapy regimens tailored to individual patients' conditions and responses.
3. Predicting Patient Outcomes
Machine learning systems can predict which patients are at risk for certain conditions, allowing for early intervention. This predictive capability is essential for managing chronic diseases.
4. Drug Discovery
Machine learning accelerates the drug discovery process by analyzing the interactions of chemical compounds and biological data to identify potential new drugs more efficiently.
Examples of Medical Datasets Used in Machine Learning
Numerous public and proprietary datasets contribute to the advancement of machine learning in healthcare:
- PhysioNet: A repository of complex biomedical datasets, including those relevant to ICU monitoring.
- MIMIC-III: A freely accessible critical care database that includes de-identified health data of thousands of patients.
- TCGA (The Cancer Genome Atlas): A comprehensive resource for cancer genomics data, covering multiple cancer types.
- Kaggle Datasets: Kaggle hosts numerous datasets, including challenges related to breast cancer detection and diabetic retinopathy.
The Future of Medical Datasets in Machine Learning
The future promises even more sophisticated applications of machine learning in healthcare, driven by advancements in big data analytics, computational power, and artificial intelligence (AI). The possibilities include:
- Real-time Analytics: The ability to analyze patient data as it is gathered will enhance immediate clinical decisions.
- Wider Accessibility of Data: Efforts to standardize and anonymize medical data will lead to broader availability for research and algorithm training.
- Interdisciplinary Collaboration: Partnerships between healthcare professionals, data scientists, and technologists will foster innovation in ML applications.
How Keymakr.com Integrates Technology in Home Services
While the primary focus of this article is on the utilization of medical datasets for machine learning, businesses like Keymakr.com are also leveraging technology to enhance services in different arenas, including Home Services and Keys & Locksmiths. By integrating advanced technologies and data analytics, they offer:
- Smart Lock Solutions: Enhancing security with locks that can be monitored and controlled via smartphone apps.
- Convenient Key Duplication Services: Utilizing technology for seamless ordering and delivery of key duplication.
- Home Automation Systems: Implementing systems that integrate locks, alarms, and cameras for better home security.
Conclusion: Bridging the Gap Between Medicine and Technology
The intersection of healthcare and technology through the utilization of medical datasets for machine learning is not just a trend; it is a transformative movement shaping the future of health services. With ongoing advancements, the healthcare industry will continue to leverage these datasets to enhance patient care, optimize processes, and drive innovation. As reflected in the innovative approaches of companies like Keymakr.com, technology's role in our everyday lives is becoming increasingly pivotal—marking an era of enhanced safety and service across diverse industries.
Further Exploration and Learning
For those interested in delving deeper into machine learning's impact on healthcare, here are some resources to consider:
- Scientific Articles on ML in Healthcare
- Kaggle Datasets Repository
- PhysioNet Resource
- Cancer Genome Atlas